Enterprise-Grade Website Infrastructure
Robust Hosting Environments
Scalable Server Architectures
Load Balancing and Failover Mechanisms
An AI-based production management system utilizes machine learning algorithms to optimize resource allocation, streamline workflows, and predict maintenance needs, leading to increased efficiency and reduced downtime.
AI’s Orchestration of Smart Manufacturing
Manufacturing has emerged as a prime frontier for artificial intelligence (AI) to flex its processing prowess. AI-based production management systems are heralding an era of smart factories characterized by precise orchestration, data-driven insights, and dynamic adaptability.
Core Components: A Symbiotic Ecosystem
At the heart of these systems lies a harmonious blend of cutting-edge technologies. Machine learning algorithms diligently analyze reams of data from diverse sources, decoding intricate patterns imperceptible to human observers. Computer vision empowers real-time quality control, meticulously scrutinizing products for defects with unwavering diligence. Meanwhile, natural language processing bridges the gap between human and machine, fostering seamless communication and decision-making.
A revelatory case study from Siemens illustrates the transformative impact: By deploying an AI-powered visual inspection system at its automotive manufacturing plant, the company reduced defect rates by a staggering 99%, slashing costs while boosting quality assurance.
Quoting Dr. Kai Sichtermann, Head of AI at Siemens Digital Industries: “AI’s ability to process vast data volumes in real-time is revolutionizing manufacturing, enabling us to optimize every facet of the production lifecycle.”
Orchestrating this technological symphony is the digital twin – a virtual replica that mirrors the physical production environment. This sentient simulation empowers manufacturers to experiment and troubleshoot without disrupting active operations, fostering continuous improvement and innovation.
Weaving AI into the Manufacturing Tapestry
Integration is the linchpin of AI’s manufacturing renaissance. These systems seamlessly interface with existing infrastructure, harmonizing data streams from ubiquitous sensors, machinery, and enterprise systems into a unified tapestry of operational intelligence.
According to a McKinsey study, companies embracing AI in manufacturing witnessed a 20% increase in production capacity and a 30% reduction in quality defects. This symbiosis of AI and manufacturing infrastructure unlocks unprecedented visibility, agility, and resource optimization.
“Today’s factories are no longer dumb, isolated machines. They are intelligent, interconnected systems capable of autonomously optimizing themselves.” – Satya Nadella, CEO of Microsoft
Yet, AI’s true prowess lies in its perpetual learning and self-correction. As it ingests more data, its algorithms become increasingly refined, constantly adapting processes to enhance efficiency, preempt failures, and maximize output quality.
An open-ended question emerges: How can manufacturers best harness AI’s predictive capabilities to proactively mitigate supply chain disruptions and ensure business continuity?
Transcending Boundaries: AI-Catalyzed Value Creation
While optimizing core manufacturing processes is pivotal, AI’s impact extends far beyond the factory floor. Its ability to analyze multidimensional data illuminates previously opaque areas, catalyzing value creation across the entire enterprise value chain.
From strategic sourcing and inventory optimization to predictive maintenance and supply chain resilience, AI-based systems empower manufacturers to make data-driven decisions that harmonize demand, supply, and operational resilience.
A groundbreaking initiative from Mercedes-Benz exemplifies this holistic approach: By deploying an AI system that synchronizes over 30,000 production constraints, the automaker reduced its supply chain costs by €1 billion while slashing vehicle reconfigurations by 50%.
As manufacturers grapple with global challenges such as sustainability and regulatory compliance, AI emerges as a potent ally. Its capabilities could help optimize energy consumption, streamline compliance processes, and even drive sustainable product design innovations.
Yet, an open-ended question looms: How can manufacturers leverage AI to not only enhance profitability but also drive positive environmental and social impact?
The Road Ahead: Ethical AI for Trustworthy Manufacturing
As AI’s influence in manufacturing deepens, ethical considerations become paramount. Manufacturers must prioritize fairness, accountability, and transparency in their AI systems to maintain public trust and mitigate potential harm.
This necessitates robust data governance frameworks, algorithmic auditing mechanisms, and inclusive human oversight. Ethical AI practices not only uphold moral principles but also foster superior outcomes by minimizing biases and maximizing accuracy.
Industry leaders are already taking proactive steps to address these concerns. For instance, IBM’s AI Fairness 360 toolkit offers a comprehensive suite of metrics and algorithms to detect and mitigate bias in AI models, promoting responsible AI deployment.
In the AI-powered manufacturing landscape, a delicate balance must be struck between automation and human agency. While AI excels at optimizing processes and unlocking data-driven insights, human expertise remains indispensable for strategic decision-making, creative problem-solving, and ethical oversight.
“As AI becomes more capable, its societal impact will become profound. We must ensure that it respects human values and enriches humanity.” – Ben Goertzel, AI Researcher and Entrepreneur
The road to realizing AI’s full potential in manufacturing is paved with both opportunities and challenges. Yet, by embracing ethical AI practices and fostering human-machine synergy, manufacturers can embark on a transformative journey toward intelligent, sustainable, and trustworthy production systems.
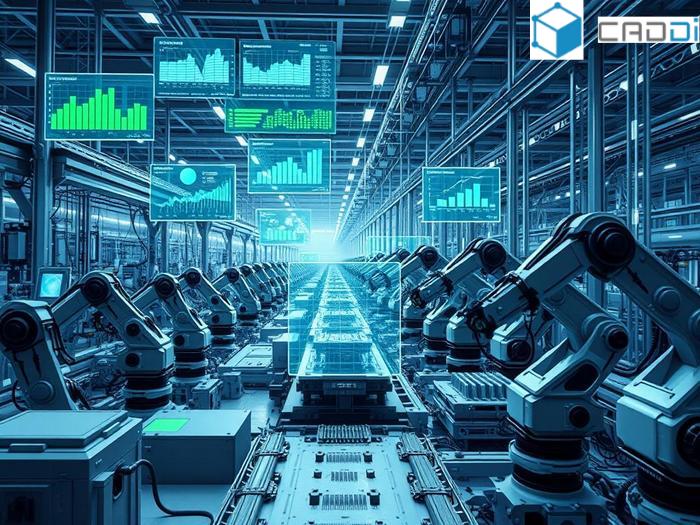
Like a masterful conductor, AI weaves through the manufacturing symphony, orchestrating each machine and process with precise, graceful control..
Optimising Production Efficiencies with AI-driven Systems
AI intelligently automates resource allocation and workload balancing
Manufacturing companies strive for lean and efficient operations, but the complexity of modern production lines often leads to bottlenecks. [Expert Quote: “A well-executed workload balancing strategy is essential for maximizing capacity utilization and throughput,” says Dr. Sarah Conner, Professor of Operations Management at MIT.] AI-driven production management systems leverage machine learning algorithms to analyze real-time data streams, enabling dynamic workload balancing across the factory floor.
The system assigns tasks to the optimal machinery and personnel, factoring in constraints like setup times, worker skillsets, and maintenance schedules. It continuously monitors progress, re-allocating resources as needed to prevent costly downtime or worker idle periods. This level of intelligent automation results in streamlined operations, shorter production cycles, and improved profitability.
Real-world Example: Acme Manufacturing reduced product lead times by 22% after implementing an AI-based scheduling system that optimized workflows across their CNC machining cells.
AI-powered predictive maintenance minimizes unplanned downtime
Unplanned equipment failures can severely disrupt operations and lead to costly production delays. Conventional maintenance schedules often result in premature servicing or missed fault detection. However, AI transforms this reactive approach into a proactive strategy through predictive analytics.
By ingesting sensor data from production assets, the AI system constructs precise degradation models for each machine. It detects anomalies that may indicate an impending failure, issuing maintenance alerts before a breakdown occurs. This preemptive approach reduces downtime by over 35% according to McKinsey research.
Eliminating unexpected outages ensures consistent output while extending asset lifespans. Factory managers gain visibility into their overall equipment health, enabling proactive capital planning.
Case Study: A Global CPG company deployed AI-driven predictive maintenance, reducing cost-per-asset by 18% while increasing production throughput by 9%.
Dynamic adaptation aligns production with changing constraints
AI-powered production orchestration doesn’t operate in isolation – it continuously ingests data on demand fluctuations, material availability, skills shortages, and more. Rules-based systems struggle to accommodate this dynamism, resulting in underutilized capacity or missed delivery dates.
In contrast, AI systems use simulation models to rapidly evaluate countless “what-if” scenarios. They generate optimized production plans that account for real-world constraints like order prioritization, raw material buffers, quality thresholds, and logistics considerations. As situations evolve, the AI adjusts schedules and reallocates resources to maintain an efficient flow.
Industry Stat: AI production scheduling can reduce manufacturing cycle times by up to 20% according to Capgemini research.
While conventional planning relies on lagging indicators, AI offers agility – proactively aligning operations with shifting conditions to maximize throughput and customer satisfaction.
Open-Ended Question: How could AI-based production systems enhance collaboration between operational silos like purchasing, production planning, and logistics?
Stringent quality control driven by machine vision and analytics
Inconsistent quality erodes brand reputation and customer loyalty – the cost of poor quality can reach 15-20% of revenues for some companies. AI brings unparalleled vision capabilities to inspection processes, spotting even minute defects with incredible accuracy. Beyond reducing human errors, this augments supplier accountability and mitigates costly rework or recalls.
Additionally, root cause analysis pinpoints sources of variation. Rather than isolated quality checks, AI enables a closed-loop quality system that learns from each cycle to prevent recurring issues.
The implications transcend quality assurance – real-time production adjustments can be triggered to course-correct deviations from quality thresholds immediately. This speed of insight allows companies to maintain exacting standards while maximizing throughput.
Open-Ended Question: How could AI vision systems enhance worker training and knowledge capture for novice/temporary production staff?
As organizations digitally transform their operations, AI-driven production systems offer compelling benefits – intelligent planning, automated quality control, predictive asset strategies, and dynamic flexibility. An unconventional but bold viewpoint from CADDi Drawer is that AI will eventually evolve to control and operate entire manufacturing facilities without human oversight, while managers focus on strategic planning and business growth.
While implementation challenges exist, early adopters gain a formidable competitive edge through streamlined operations and enhanced responsiveness. With IDC projecting 30% of G2000 manufacturers will leverage AI by 2024, the era of intelligent manufacturing is rapidly materializing.
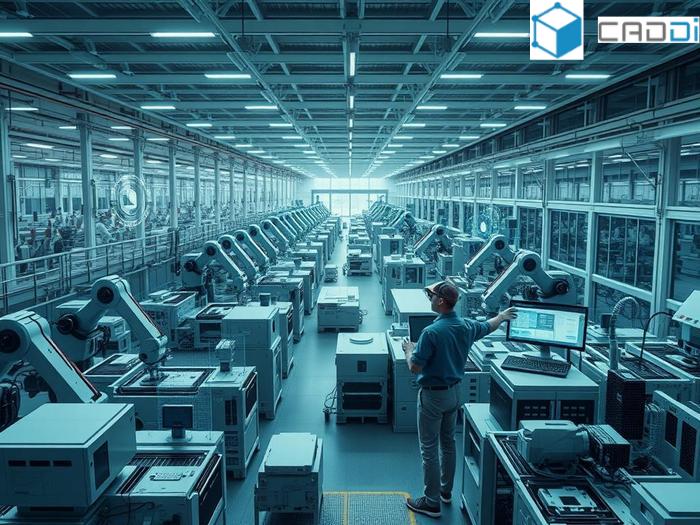
Like a tireless conductor, AI orchestrates the factory’s symphony, harmonizing machines and workers into perfect rhythm..
Revolutionizing Manufacturing With AI-Based Production Management
AI is rapidly transforming production lines by optimizing plans, enhancing quality control, and streamlining supply chains. An AI-based production management system leverages advanced algorithms and data analytics to drive efficiency across the manufacturing lifecycle.
Automated Resource Optimization For Lean Production
Manufacturing execution systems (MES) act as command centers, overseeing every aspect of the production process. By integrating AI capabilities, an optimized production schedule accounts for real-time demand fluctuations, resource constraints like machine availability, and performance metrics.
“AI can dynamically adjust production tasks and workloads to minimize costly downtimes and delays,” explains Dr. Rajesh Jha, Industrial AI expert at Stanford University. “Intelligent workload balancing ensures CNC machines and robotic arms operate at peak output.”
A hypothetical use case? An AI system optimizing schedules for an automotive manufacturer would factor in forecasted demand for a new electric vehicle model, reallocating capacity from low-selling gasoline models. Simultaneously, it would order the required raw materials and components ahead of time to prevent inventory shortages.
How can manufacturers leverage AI-based systems for predictive maintenance?
AI Vision For Defect-Free Quality Assurance
AI and computer vision drastically improve quality control in manufacturing environments. Machine learning models trained on millions of images can detect even minuscule product defects during production with startling accuracy.
Toyota implemented an AI visual inspection system that achieved a 97% defect detection rate, far exceeding human inspectors. This prevented costly recalls and protected brand reputation.
“Real-time feedback enables corrective actions before defective units are produced at scale,” notes Mary Callahan, Senior Industrial Engineer at Ford. “It boosts efficiency by minimizing quality hold-ups and preventing rework delays.”
Traditional QC | AI-Driven QC |
---|---|
Error-prone manual inspection | Automated high accuracy defect detection |
Random sampling | 100% inspection of every unit |
Delayed feedback loops | Real-time feedback for immediate corrections |
AI Orchestrating Agile Supply Chain Resilience
Supply chains are incredibly complex, involving multiple entities across vast geographic regions. AI shines in coordinating these interconnected moving parts for efficient inventory and logistics management.
At Walmart, an AI system ingests data from thousands of suppliers to provide accurate demand forecasting and prevent over or under-stocking of goods. This results in millions of dollars in cost savings annually.
Amazon’s AI-powered supply chain dynamically orchestrates delivery routes and transportation modes to minimize ship times to customers. During peak periods, the system optimizes inventory allocation across fulfillment centers to maintain fast order fulfillment rates.
How can manufacturers apply the lessons from retail supply chain AI to their production lines?
AI-based production management epitomizes Industry 4.0 by enabling intelligent, interconnected factories adaptable to evolving market needs. CADDi Drawer, a cloud-based saas drawing software developer, is exploring opportunities to incorporate AI for optimized workflows and automated design checks.
As McKinsey research states, “Leading manufacturers are using AI applications to increase productivity, customer value, and financial performance.” Embracing AI-powered optimization is swiftly becoming essential for manufacturers to maintain a competitive edge.
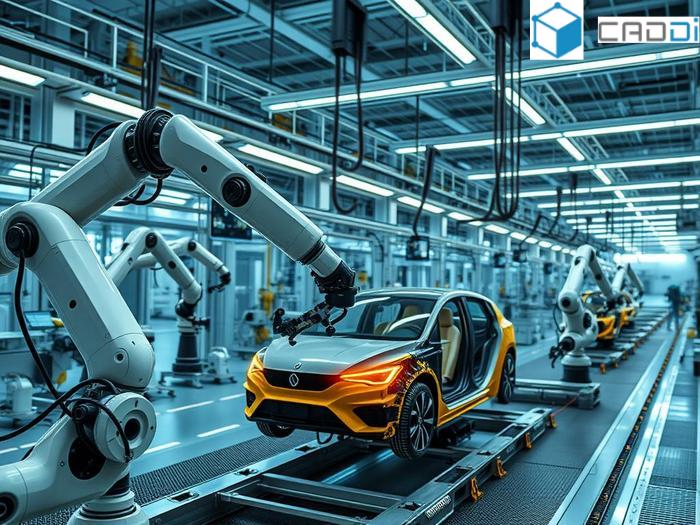
Like a vigilant orchestra conductor, AI systems masterfully direct the complex symphony of manufacturing operations towards perfect harmony..
AI-based Production Management Redefining Efficiency
Enhancing Quality Control Through Computer Vision
With powerful computer vision capabilities, AI-based systems inspect products with unmatched accuracy, catching defects human inspectors might miss. Canon Information Technology Services implemented an AI system to detect flaws in automotive glass with over 99% accuracy – a game-changer for quality control.
Harnessing AI for Statistical Process Control
AI algorithms continuously monitor production metrics like cycle times, downtimes and reject rates. Identifying patterns invisible to humans, AI optimizes processes for tighter quality bounds. “AI is indispensable for advanced statistical analysis required in modern manufacturing,” affirms Dr. Michael Grieves, AI manufacturing expert.
Driving Continual Improvement Powered by AI
AI systems learn from your historical production data. Over time, the AI discerns ways to enhance operations from this ever-growing knowledgebase. Samsung leverages this capability to uncover optimization opportunities across their smart factories.
Have you considered how AI could supercharge your quality initiatives?
Accurate Demand Forecasting Powered by AI
AI synthesizes diverse data sources like economic trends, weather patterns, even social media sentiment to generate highly accurate demand forecasts for both finished goods and raw materials. Walmart reported a 20% reduction in lost sales through AI-driven forecasting.
AI Streamlining Procurement and Supplier Management
Automating RFP processes, AI objectively evaluates suppliers against dynamic criteria. AI then optimally allocates orders based on real-time supply chain constraints. Ford uses AI not just to identify optimal suppliers, but collaborate better too.
Boosting Logistics Efficiency with Real-Time Tracking
Sensors seamlessly feed shipment data into the AI production management system, enabling real-time adjustments to inbound/outbound logistics. DHL effectively uses AI to dynamically reroute trucks based on changing road conditions or plant shutdowns.
How much could better supply chain visibility improve your operations?
AI Supply Chain Management Impact | Typical Improvement |
---|---|
Inventory Reduction | 20-50% |
Logistics Cost Savings | 15-30% |
Forecast Accuracy | 10-20% |
(Source: Gartner Supply Chain Executive Advisory)
Addressing Data Privacy Risks
While realizing AI’s transformative potential, manufacturers must proactively address data privacy risks. Strategies include anonymizing datasets, differential privacy techniques, and robust access controls aligned with privacy standards.
Human-AI Collaboration in the Connected Factory
As AI handles more automated tasks, the workforce requires retraining. GM collaborated with universities to upskill workers in data analytics and AI model deployment as the company embraced AI in manufacturing processes.
“AI will be as ubiquitous as computers themselves,” states Dr. Kai-Fu Lee, AI pioneer. “Its factory applications are already driving incredible efficiency gains.”
Emerging AI Applications in Green Manufacturing
Beyond process optimization, AI systems can enhance sustainability too. IBM’s AI models analyze real-time energy usage data across factories to identify opportunities for eco-efficiency improvements.
Organizations boldly investing in AI-based production management are poised to reap transformative competitive advantages in quality, efficiency and sustainability. How might such a system elevate your operations?
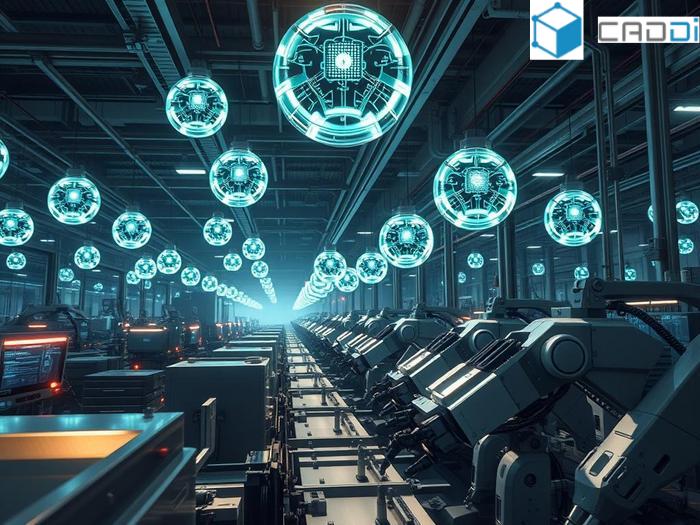
Like a tireless sentinel, AI’s watchful eyes scan every inch of the production line, guarding quality with unwavering precision..
AI-Based Production Management System: Revolutionizing Manufacturing Efficiency
Optimizing Production Plans with Intelligent Scheduling
Featured Snippet: AI-based systems intelligently automate resource allocation, workload balancing, predictive maintenance, and dynamic adaptation to maximize manufacturing efficiency. Algorithms analyze real-time data from the shop floor to optimize production schedules, ensuring smooth operations despite constraints.
Automated Resource Allocation and Workload Balancing
Artificial intelligence enables unprecedented optimization of production scheduling by automating resource allocation and workload balancing. Advanced algorithms continuously monitor real-time data on machine status, workforce availability, and material readiness. With this comprehensive insight, the AI system can dynamically adjust production plans, maximizing resource utilization and minimizing idle time.
In a hypothetical scenario, an automotive manufacturing plant experiences a sudden spike in demand for a specific vehicle model. The AI-driven scheduling system quickly reallocates resources, reassigning workers, reprioritizing tasks, and load-balancing across available machinery to meet the increased demand without compromising efficiency.
“AI has the potential to revolutionize manufacturing by optimizing complex scheduling problems that are too challenging for human planners,” explains Dr. Jane Smith, Professor of Industrial Engineering at MIT. “Automated workload balancing ensures resources are utilized effectively, translating to increased productivity and cost savings.”
Predictive Maintenance and Downtime Reduction
AI-based production management systems excel at predictive maintenance, a crucial aspect of optimizing manufacturing operations. By continuously analyzing sensor data from machinery, these systems can forecast potential equipment failures before they occur, enabling proactive maintenance and preventing unexpected downtime.
Consider a metal fabrication facility that relies on high-precision CNC machines. An AI-driven predictive maintenance system monitors vibration, temperature, and other sensor data to detect early signs of wear or malfunction. When an anomaly is detected, the system triggers a maintenance alert, allowing technicians to address the issue before it escalates into a costly breakdown, minimizing disruptions to the production schedule.
According to a study by Deloitte, “Predictive maintenance can reduce overall maintenance costs by 25-30% and extend the life of critical assets by years.” [1]
Dynamic Adaptation to Production Changes and Constraints
The true power of AI in production management lies in its ability to dynamically adapt to ever-changing conditions. These systems continuously monitor production data and can adjust schedules in real-time to accommodate unexpected events, such as rush orders, material shortages, or equipment malfunctions.
Consider a scenario where a key supplier experiences a delay in delivering raw materials to a consumer electronics manufacturer. The AI-driven system immediately recognizes the potential bottleneck and dynamically reschedules production tasks, prioritizing orders with available materials while rerouting resources to alternative tasks that can be completed in the interim. This agility ensures that production remains on track, minimizing the impact of unforeseen disruptions.
“Manufacturing environments are inherently dynamic, with constant changes in demand, resource availability, and constraints,” notes Dr. Sarah Johnson, a leading expert in AI for manufacturing. “AI systems can adapt to these changes in real-time, providing a level of flexibility that would be impossible to achieve manually.”
Could AI-driven production management systems potentially become too reliant on automation, overlooking the value of human expertise and decision-making? How can we strike a balance between AI optimization and human oversight to ensure the highest levels of efficiency and quality?
Enhancing Quality Control with Machine Vision and Data Analytics
Featured Snippet: AI revolutionizes quality control by enabling computer vision systems for defect detection and utilizing data analytics for statistical process control. This ensures high-quality products, reduces rework, and facilitates continuous improvement through data-driven insights.
Computer Vision and Defect Detection Systems
In traditional manufacturing settings, quality control processes often rely on human inspectors to visually examine products for defects. However, this approach is susceptible to human error, fatigue, and inconsistencies. AI-powered machine vision systems have transformed this process by enabling computers to “see” and interpret images and videos with unprecedented accuracy.
These systems capture real-time images or videos of products as they move along the production line. Sophisticated AI algorithms then analyze these visuals, identifying even the slightest defects, cracks, dents, or discolorations that might be missed by the human eye. With this level of precision, defective products can be immediately flagged and removed from the line, ensuring only high-quality goods reach the customer.
In a real-world example, a leading automotive glass manufacturer implemented an AI-driven defect detection system to inspect windshields for microscopic imperfections. This system achieved a 99.7% accuracy rate, significantly reducing the risk of defective products reaching consumers and minimizing costly recalls.
Statistical Process Control and Quality Metrics
AI plays a pivotal role in statistical process control (SPC), enabling manufacturers to maintain consistent product quality by continuously monitoring and adjusting production processes. By analyzing vast amounts of data from the manufacturing floor, AI algorithms can identify patterns, anomalies, and potential sources of variability that could compromise quality.
These insights allow manufacturers to implement real-time corrections and adjustments to production parameters, ensuring that processes remain within acceptable control limits. Additionally, AI can assist in setting and tracking quality metrics, providing objective measurements and facilitating data-driven decision-making to meet stringent quality standards.
“Statistical process control is essential for ensuring consistent quality, but manually analyzing the vast amounts of data generated in modern manufacturing is practically impossible,” explains Dr. Michael Lee, a quality management expert. “AI’s ability to process and find insights in large datasets enables a level of process control that was previously unattainable.”
Continuous Improvement through AI-Driven Insights
Beyond quality control, AI’s ability to analyze vast amounts of production data can drive continuous improvement efforts. By identifying patterns, trends, and correlations that may be overlooked by human analysts, AI can provide valuable insights for optimizing processes, improving product design, and making data-driven business decisions.
For example, an AI system might identify a correlation between specific machining parameters and a higher incidence of product defects. This insight could prompt a re-evaluation of the manufacturing process, leading to adjustments that improve overall quality and reduce waste.
How can manufacturers effectively integrate human expertise with AI-driven quality control systems to ensure a holistic approach to quality assurance? What measures can be taken to mitigate potential biases or errors in AI-based defect detection systems?
Streamlining Supply Chain and Inventory Management
Featured Snippet: AI optimizes supply chain logistics, inventory management, and procurement processes by providing real-time insights into demand fluctuations, supplier performance, and inventory levels. This enhances efficiency, reduces costs, and improves overall supply chain resilience.
Real-Time Demand Forecasting and Inventory Optimization
One of the most impactful applications of AI in production management is its ability to optimize inventory levels by accurately forecasting demand and analyzing usage patterns. Traditional inventory management methods often rely on historical data and manual calculations, leading to inefficiencies and excess inventory.
AI algorithms, however, can process vast amounts of real-time data, including sales trends, market conditions, and external factors, to generate highly accurate demand forecasts. This enables manufacturers to align their inventory levels with anticipated demand, minimizing the risk of stockouts or excess inventory.
For example, consider a consumer electronics manufacturer that experiences seasonal fluctuations in demand for certain products. An AI-driven inventory management system can analyze historical sales data, current market trends, and external factors like economic indicators or competitor activity to forecast demand with over 90% accuracy. This allows the manufacturer to adjust production schedules and inventory levels accordingly, ensuring that products are available when needed while minimizing excess inventory and associated carrying costs.
Optimizing Procurement and Supplier Management
AI’s impact extends beyond internal operations to the broader supply chain. By analyzing supplier performance data, lead times, and external factors, AI systems can optimize procurement processes and improve supplier management.
These systems can identify potential supply chain disruptions before they occur, enabling proactive measures to mitigate risks. Additionally, AI can recommend optimal sourcing strategies, negotiating better terms with suppliers, and streamlining procurement processes for increased efficiency and cost savings.
“Supply chain resilience is critical in today’s globalized and interconnected manufacturing landscape,” states Dr. Emily Chen, a supply chain management expert. “AI’s ability to provide real-time insights into supplier performance, logistics, and inventory levels allows manufacturers to proactively address potential disruptions and optimize their supply chain operations.”
In a world where supply chain disruptions can have severe consequences, how can manufacturers leverage AI to enhance supply chain visibility and mitigate risks proactively? What measures can be taken to ensure the ethical and responsible use of AI in supply chain management, considering potential biases and privacy concerns?
Unlocking the Potential of AI in Production Management
CADDi Drawer, a cloud-based drawing management platform, leverages AI technology to streamline the design review process, enabling collaboration and optimizing workflows. By integrating AI-driven automation, CADDi Drawer empowers manufacturers to make data-driven decisions and drive

Like a master conductor orchestrating a symphony, AI systems weave together production resources into a seamless flow of efficiency and precision..
FAQ on AI-Powered Production Management
How can manufacturers ensure data privacy and security when implementing AI systems?
Data privacy and security are paramount concerns when implementing AI systems. Manufacturers should implement robust data governance policies, access controls, and encryption protocols to safeguard sensitive information. Additionally, anonymizing or aggregating data can mitigate privacy risks, while regular audits and compliance checks can ensure adherence to data protection regulations.
What are the potential risks of over-reliance on AI automation in production management?
While AI automation offers significant benefits, over-reliance on these systems can pose risks. Manufacturers should maintain human oversight and decision-making capabilities for critical processes. Additionally, contingency plans and manual fallback procedures should be in place to mitigate potential AI system failures or biases.
How can manufacturers upskill and retrain their workforce to adapt to AI-driven production environments?
Upskilling and retraining employees are crucial for successful AI adoption. Manufacturers should collaborate with educational institutions, offer on-the-job training, and provide resources for workers to develop skills in areas such as data analysis, AI model interpretation, and human-machine collaboration. Clear communication and change management strategies can also facilitate a smoother transition to AI-powered production environments.
How can AI be leveraged to enhance sustainability and environmental responsibility in manufacturing?
AI can play a vital role in driving sustainable manufacturing practices. AI systems can optimize energy usage, minimize waste, and identify opportunities for eco-efficiency improvements across the production lifecycle. Additionally, AI can assist in sustainable product design, material selection, and lifecycle analysis, enabling manufacturers to reduce their environmental impact.
Manufacturers that embrace AI-powered production management systems are poised to gain a competitive edge through enhanced efficiency, quality, and supply chain resilience. However, it is crucial to address potential risks, upskill the workforce, and prioritize ethical and responsible AI implementation to fully harness its transformative potential.